Welcome to the Machine Learning Research Group at the Institute of Structural Mechanics and Dynamics in Aerospace Engineering. Our group specializes in application and methodological development of machine learning techniques in mechanics.
We are actively engaged in developing physics-informed machine learning models that integrate domain-specific knowledge to enhance the accuracy and interpretability of predictions especially in coupled problems. Additionally, our research extends into the realm of medical image analysis, where we leverage machine learning algorithms to segment and analyze medical images. The obtained insight are leveraged to enhance diagnostic capabilities and are processed to generate input for subsequent simulations.
Collaborating closely with the other research groups, we develop surrogate models that efficiently represent high-fidelity simulations, facilitating accelerated design processes, near real-time predictions, and optimization studies across various disciplines. Our expertise in regression and classification allows us to analyze experimental data and clinical datasets from us and our partners. We leverage this approach to gain deeper insights into model discovery, refinement, and simulation.
For questions, inquiries, or to express interest, please feel free to reach out to us.
Publications
2023
- Mandl, L., Mielke, A., Seyedpour, S. M., & Ricken, T. (2023). Affine transformations accelerate the training of physics-informed neural networks of a one-dimensional consolidation problem. Scientific Reports, 13(1), Article 1. https://doi.org/10.1038/s41598-023-42141-x
Head of Group
Researcher
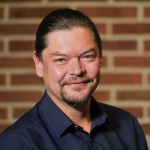
Tim Ricken
Univ.-Prof. Dr.-Ing.Head of Department