The mechanical behavior of fluid-saturated porous materials is usually described via a continuum-mechanical homogenization approach, such as the Biot theory, mixture theory or theory of porous media (TPM), since a direct discrete description of the microstructure including the involved phases and their interactions is extremely costly. Even for the simplest case of a porous biphasic body, the description leads to a system of coupled differential equations to determine the process variables of fluid pressure and solid displacement. The high numerical effort is opposed to the need of answering relevant engineering questions, such as inverse parameter identification (IPI), optimization, or uncertainty quantification (UQ), for which a high number of simulation runs is required. For this reason, the development of reduced but still accurate surrogate models (surrogates) for porous materials is required.
The development of surrogate models is based on knowledge- or data-driven approaches. Each approach is characterized by typical advantages and disadvantages. Simplifying assumptions have to be formulated for knowledge-driven methods, reducing the geometrical or physical problem complexity. The ability to perform analytical error estimation is a key advantage here. Data-driven methods, in contrast, generalize better and produce good results even in high-complexity regions, but need a large amount of high-fidelity simulation data for their highly time-consuming training. Additionally, they are naturally prone to unexpected errors in their predictions that cannot be captured properly by a priori error estimation. A further disadvantage is the nearly impossible interpretability of the models. The challenge lies in developing custom surrogates that amplify the advantages and simultaneously suppress disadvantages for their intended application (IPI, optimization, UQ).
We develop innovative model order reduction methods (MOR) for biphasic porous materials, which maintain a reasonable balance between knowledge- and data-driven approaches. A key feature that will be utilized for knowledge-driven MOR is that many porous materials can be (partially) considered thin materials with a geometrical scale separation. Based on this, the method of asymptotic expansions will be used to derive knowledge-driven reduced models. In addition, several data-driven models, using deep learning techniques, will be developed. Among the promising candidates for data-driven techniques are standard artificial neural networks and variational autoencoders. Furthermore, hybrid knowledge- and data-driven MOR approaches, such as physics-informed neural networks and custom combinations of the pure techniques, will be designed for nonlinear materials with mixed-type complex geometries. Finally, all models will be validated and tested for robustness and computational efficiency for IPI, optimization, and UQ.
Publications
2021
- Armiti-Juber, A., & Ricken, T. (2021). Model order reduction for deformable porous materials in thin domains via asymptotic analysis. Archive of Applied Mechanics, 92(2), Article 2. https://doi.org/10.1007/s00419-021-01907-3
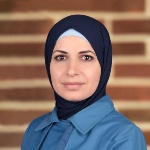
Alaa Armiti-Juber
Dr. rer. nat.Head of Model Order Reduction & Numerics Group, Researcher