Physics-based modeling has been the classical tool for describing and predicting the behavior of multiphysical systems. High fidelity simulations of such systems require suitable numerical schemes as well as fine scale spatio-temporal grids.
Within multiphysics, the proper design of such numerical schemes is challenging. Beside stability, even in the asymptotic ranges of the material parameters, accuracy of primal (e.g., displacement) as well as dual quantities (e.g., stresses) is required. Within many engineering applications accurate dual quantities are even more relevant than the primal ones. Strictly speaking, this requires the incorporation of primal as well as dual quantities into the numerical schemes, enlarging size and complexity of the emerging linear equation systems. In return, this paves the way to construct parameter-robust, adaptive solution strategies, delivering guaranteed accuracy on tailored spatio-temporal grids.
Even with the current advances of computational capabilities, many engineering questions, such as optimization, uncertainty quantification, model-based predictive control or clinical-time constraints require a reduction of computational complexity. Therefore, reconstruction-based techniques, such as equilibration, reduces complexity by calculating sufficiently accurate fluxes based on the primal variables. A direct incorporation into the numerical scheme is therefore not required. A different approach dealing with the same issue is model-order reduction (MOR).
MOR methods have been developed to reduce computational time complex, multiphysical systems while preserving their important physical features. Most popular MOR methods can be classified into three main categories: 1) knowledge-driven approaches utilizing tools such as asymptotic analysis and homogenization. 2) Projection-based methods extracting the essential dynamical features and projecting the models onto those features. 3) Data-driven approaches applying machine learning methods on simulation or experimental data.
In this group we investigate the applicability of different MOR methods and reconstruction-based techniques on different industrial, medical, and environmental applications. Moreover, novel model-reduction methods, based hybrid reduction and reconstruction techniques, will be developed and tested. Our aim is to achieve the most possible fast predictions, while still maintaining acceptable stability and accuracy levels.
Publications
2025
- Armiti-Juber, A., & Ricken, T. (2025). A multigrid two-scale modeling approach for nonlinear multiphysical systems. Computer Methods in Applied Mechanics and Engineering, 433, 117523. https://doi.org/10.1016/j.cma.2024.117523
2024
- Brodbeck, M., Egli, F. S., Suditsch, M., Seyedpour, S. M., & Ricken, T. (2024). On the influence of non-linearity within two-phase poro-elasticity: Numerical examples and counterexamples. Examples and Counterexamples, 6. https://doi.org/10.1016/j.exco.2024.100167
- Brodbeck, M., Bertrand, F., & Ricken, T. (2024). Adaptive finite element methods based on flux and stress equilibration using FEniCSx. https://arxiv.org/abs/2410.09764
- Brodbeck, M., Suditsch, M., Seyedpour, S. M., & Ricken, T. (2024). Phase transition in porous materials: effects of material parameters and deformation regime on mass conservativity. Computational Mechanics. https://doi.org/10.1007/s00466-024-02557-2
2023
- Bertrand, F., & Brodbeck, M. (2023). Adaptive strategies based on equilibration for the Biot equations. Pamm, 23, Article 1. https://doi.org/10.1002/pamm.202200240
- Soltani, K., Seyedpour, S. M., Ricken, T., & Rezazadeh, G. (2023). Transient high-frequency spherical wave propagation in porous medium using fractional calculus technique. Acta Mechanica. https://doi.org/10.1007/s00707-023-03780-3
- Arasteh-Khoshbin, O., Seyedpour, S. M., Brodbeck, M., Lambers, L., & Ricken, T. (2023). On effects of freezing and thawing cycles of concrete containing nano-SiO2 : experimental study of material properties and crack simulation. Scientific reports, 13, 22278. https://doi.org/10.1038/s41598-023-48211-4
2022
- Armiti-Juber, A., & Ricken, T. (2022). Model order reduction for deformable porous materials in thin domains via asymptotic analysis. Archive of Applied Mechanics, 92, Article 2. https://doi.org/10.1007/s00419-021-01907-3
- Bertrand, F., & Brodbeck, M. (2022). Robust discretizations for poroelastic problems: engineering and mathematical points of views in a presenation in pairs. The 8th European Congress on Computational Methods in Applied Sciences and Engineering. https://doi.org/10.23967/eccomas.2022.236
- Bertrand, F., Brodbeck, M., & Ricken, T. (2022). On robust discretization methods for poroelastic problems: Numerical examples and counter-examples. Examples and Counterexamples, 2, 100087. https://doi.org/10.1016/j.exco.2022.100087
2021
- Armiti-Juber, A. (2021). On the limit of a two-phase flow problem in thin porous media domains of Brinkman type. Mathematical Methods in the Applied Sciences. https://doi.org/10.1002/mma.7940
- Armiti-Juber, A., & Ricken, T. (2021). Model order reduction for deformable porous materials in thin domains via asymptotic analysis. Archive of Applied Mechanics, 92, Article 2. https://doi.org/10.1007/s00419-021-01907-3
2020
- Armiti-Juber, A., & Rohde, C. (2020). On the Well-posedness of a Nonlinear Fourth-Order Extension of Richards’ Equation. J. Math. Anal. And Appl., 487, Article 2. https://doi.org/10.1016/j.jmaa.2020.124005
2019
- Armiti-Juber, A., & Rohde, C. (2019). Existence of weak solutions for a nonlocal pseudo-parabolic model for Brinkman two-phase flow in asymptotically flat porous media. J. Math. Anal. And Appl., 477, Article 1. https://doi.org/10.1016/j.jmaa.2019.04.049
2018
- Armiti-Juber, A., & Rohde, C. (2018). On Darcy- and Brinkman-type models for two-phase flow in asymptotically flat domains. Computat. Geosci. https://doi.org/10.1007/s10596-018-9756-2
2014
- Armiti-Juber, A., & Rohde, C. (2014). Almost parallel flows in porous media, Finite Volumes for Complex Applications VII-Elliptic, Parabolic and Hyperbolic Problems. In J. Fuhrmann, M. Ohlberger, & C. Rohde (Eds.), Finite Volumes for Complex Applications VII-Elliptic, Parabolic and Hyperbolic Problems: FVCA 7, Berlin, June 2014 (pp. 873–881). Springer International Publishing. https://doi.org/10.1007/978-3-319-05591-6_88
Head of Group
Researchers
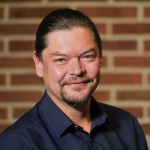
Tim Ricken
Univ.-Prof. Dr.-Ing.Head of Department