The numerical simulation of real physical processes is characterized by a complex interplay of factors such as model accuracy, physical understanding, and valid data. These challenges become particularly evident in the context of the complexity of multiscale and multiphysical modeling, as required in fields such as biology or environmental engineering. Uncertainty quantification (UQ) provides a means to analyze and assess the effects of uncertainties on the predictive accuracy of such models. It contributes to a better understanding of the reliability and significance of simulation results. These uncertainties can be addressed through an analysis that encompasses both aleatoric and epistemic uncertainties, i.e. polymorphic uncertainty quantification (PUQ), thereby accounting for variations in model input parameters as well as the challenges arising from model inaccuracies or unmeasurable elements due to incomplete knowledge. To achieve this, we employ approaches such as Bayesian and fuzzy techniques. Furthermore, the combination of optimization and uncertainty quantification offers an opportunity not only to enhance the models themselves but also to optimize the underlying assumptions and data foundations. This enables a holistic approach to complex systems and supports the development of more robust and precise models.
In both PUQ and Structural Optimization, sensitivity analysis plays a pivotal role. It aids in comprehensively assessing the influence of input parameters on a model and can also increase the efficiency of models themselves. For instance, sensitivities can be applied to create more efficient metamodels in the framework of PUQ, e.g. Gardient enhanced Kriging Model. Moreover, the integration of sensitivity analysis can lead to efficiency improvements in optimization algorithms.
Publications
2025
- Nicolas, G., Lars, K., Navina, W., & Tim, R. (2025). Shape Optimization of auxetic unit cells under dynamic loading in macroscopic components. In M. Andre & S. Afzal (eds.), AreoBest 2025 III ECCOMAS Thematic Conference on Multidisciplinary Design Optimization of Aerospace Systems Proceedings (No. 1; Vol. 1, pp. 37–51). IDMEC Instituto Superior T´ecnico Universidade de Lisboa Portugal. https://aerobest.idmec.tecnico.ulisboa.pt/wp-content/uploads/2025/05/AeroBest2025_proceedings.pdf
- Grünfelder, N., Kälber, L., Waschinsky, N., & Ricken, T. (2025). Shape Optimization of auxetic unit cells under dynamic loading in macroscopic components. In M. Andre & S. Afzal (eds.), AreoBest 2025 III ECCOMAS Thematic Conference on Multidisciplinary Design Optimization of Aerospace Systems Proceedings (No. 1; Vol. 1, pp. 37–51). IDMEC Instituto Superior T´ecnico Universidade de Lisboa Portugal. https://aerobest.idmec.tecnico.ulisboa.pt/wp-content/uploads/2025/05/AeroBest2025_proceedings.pdf
2024
- Grünfelder, N., Savall, B. P., Seyedpour, S. M., Waschinsky, N., & Ricken, T. (2024). Exploring the dependencies of Poisson’s ratio in auxetic structures. In M. Kaliske (Ed.), PAMM. Wiley. https://doi.org/10.1002/pamm.202400073
- Grünfelder, N., Savall, B. P., Seyedpour, S. M., Waschinsky, N., & Ricken, T. (2024). Exploring the dependencies of Poisson’s ratio in auxetic structures. Pamm. https://doi.org/10.1002/pamm.202400073
- Maike, S., Schröder, J., Bluhm, J., & Ricken, T. (2024). A mesh--in--element method for the theory of porous media. International Journal for Numerical Methods in Engineering. https://doi.org/10.1002/nme.7565
- Pi Savall, B., Seyedpour, S. M., & Ricken, T. (2024). Experimental Analysis of Strain and Thermal Behaviour on 3D Printed Flexible Auxetic Structures. In H. Altenbach, L. Hitzler, M. Johlitz, M. Merkel, & A. Öchsner (Eds.), Lectures Notes on Advanced Structured Materials 2 (pp. 85–102). Springer Nature Switzerland. https://doi.org/10.1007/978-3-031-49043-95
2023
- Soltani, K., Seyedpour, S. M., Ricken, T., & Rezazadeh, G. (2023). Transient high-frequency spherical wave propagation in porous medium using fractional calculus technique. Acta Mechanica. https://doi.org/10.1007/s00707-023-03780-3
- Mandl, L., Mielke, A., Seyedpour, S. M., & Ricken, T. (2023). Affine transformations accelerate the training of physics-informed neural networks of a one-dimensional consolidation problem. Scientific reports, 13, 15566. https://doi.org/10.1038/s41598-023-42141-x
- Seyedpour, S. M., Henning, C., Kirmizakis, P., Herbrandt, S., Ickstadt, K., Doherty, R., & Ricken, T. (2023). Uncertainty with Varying Subsurface Permeabilities Reduced Using Coupled Random Field and Extended Theory of Porous Media Contaminant Transport Models. Water, 15, Article 1. https://doi.org/10.3390/w15010159
2022
- Ricken, T., Schroeder, J., Bluhm, J., Maike, S., & Bartel, F. (2022). Theoretical formulation and computational aspects of a two-scale homogenization scheme combining the TPM and FE2 method for poro-elastic fluid-saturated porous media. International Journal of Solids and Structures, 241, 111412. https://doi.org/10.1016/j.ijsolstr.2021.111412
- Armiti-Juber, A., & Ricken, T. (2022). Model order reduction for deformable porous materials in thin domains via asymptotic analysis. Archive of Applied Mechanics, 92, Article 2. https://doi.org/10.1007/s00419-021-01907-3
2021
- Waschinsky, N., Barthold, F.-J., & Menzel, A. (2021). Structural optimisation of diffusion driven degradation processes. Structural and Multidisciplinary Optimization, 64, 889–903. https://doi.org/10.1007/s00158-021-02900-8
2019
- Drieschner, M., Matthies, H. G., Hoang, T.-V., Rosić, B. V., Ricken, T., Henning, C., Ostermeyer, G.-P., Müller, M., Brumme, S., Srisupattarawanit, T., & others, . (2019). Analysis of polymorphic data uncertainties in engineering applications. GAMM-Mitteilungen, e201900010. https://doi.org/10.1002/gamm.201900010
- Waschinsky, N., Barthold, F.-J., & Menzel, A. (2019). Optimisation of Diffusion Driven Degradation Processes. Pamm, 19, Article 1. https://doi.org/10.1002/pamm.201900182
- Henning, C., & Ricken, T. (2019). Polymorphic Uncertainty Quantification of Computational Soil and Earth Structure Simulations via the Variational Sensitivity Analysis. Pamm, 19, Article 1. https://doi.org/10.1002/pamm.201900289
- Schmidt, A., Henning, C., Herbrandt, S., Könke, C., Ickstadt, K., Ricken, T., & Lahmer, T. (2019). Numerical studies of earth structure assessment via the theory of porous media using fuzzy probability based random field material descriptions. GAMM-Mitteilungen, 42, Article 1. https://doi.org/10.1002/gamm.201900007
- Henning, C., & Ricken, T. (2019). Transition of the variational sensitivity analysis to polymorphic uncertainty quantification to soil investigations. Advances in Engineering Materials, Structures and Systems: Innovations, Mechanics and Applications: Proceedings of the 7th International Conference on Structural Engineering, Mechanics and Computation (SEMC 2019), September 2-4, 2019, Cape Town, South Africa, 297–302. https://doi.org/10.1201/9780429426506
2018
- Henning, C., Herbrandt, S., Ickstadt, K., & Ricken, T. (2018). Combining Finite Elements and Random Fields to Quantify Uncertainty in a Multi-phase Structural Analysis. Pamm, 18, Article 1. https://doi.org/10.1002/pamm.201800333
2017
- Henning, C., & Ricken, T. (2017). Polymorphic uncertainty quantification for stability analysis of fluid saturated soil and earth structures. Pamm, 17, Article 1. https://doi.org/10.1002/pamm.201710018
Navina Waschinsky
Dr.-Ing.Head of Optimization & Uncertainty Quantification Group, Researcher
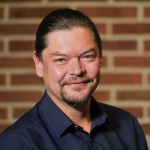
Tim Ricken
Univ.-Prof. Dr.-Ing.Head of Department